Agentic AI vs. AI Agents
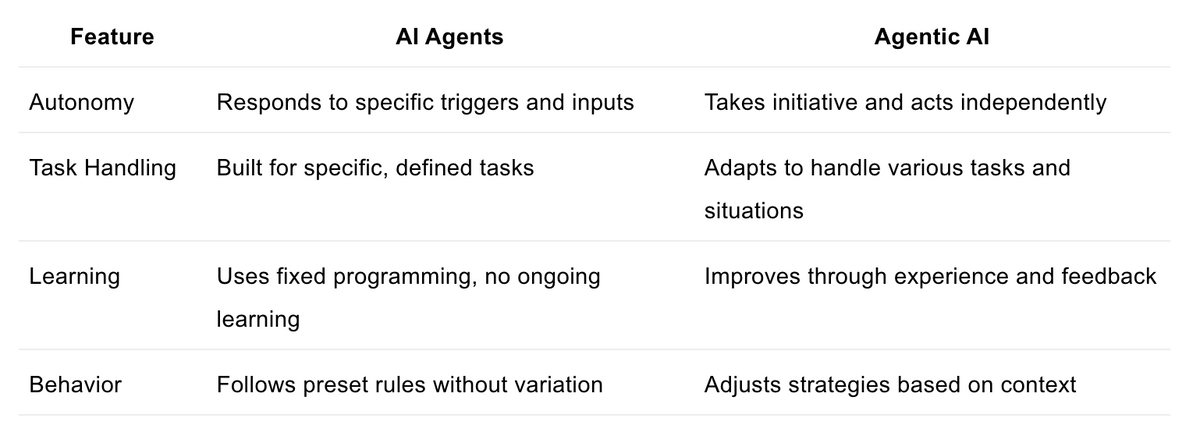
You've probably heard the terms "AI agents" and "agentic AI" in meetings or discussions. They might sound similar, but they're quite different approaches to building AI systems. Understanding these differences helps you pick the right tools for your projects.
Let's break down what sets them apart.
What are AI Agents?
AI agents are programs designed for specific tasks with clear objectives. You interact with them daily - they power customer support chatbots, handle product recommendations, and run automated scripts in backend systems.
Reactive - taking specific inputs and producing outputs based on their training. They handle defined tasks and operate within set parameters, making them ideal for structured, repetitive work.
Task-Oriented - They focus on single objectives or a limited set of related tasks. This specialization makes them efficient but also means they're not built to handle work outside their defined scope.
Limited Autonomy - This narrow focus has trade-offs. While AI agents excel at their programmed tasks, they don't adapt to new situations or learn beyond their initial training. They follow their programming strictly, which makes them reliable for specific uses but limited in flexibility.
What is Agentic AI?
Agentic AI takes automation a step further by making decisions and adapting on its own. Unlike basic AI agents, these systems can work independently, set their own goals, and change their approach based on what they learn.
What sets agentic AI apart? Three main things:
Goal-setting - The system can figure out what needs to be done without being explicitly told. Instead of waiting for instructions, it can identify goals and work toward them - like autonomous robots mapping new environments or AI assistants that anticipate user needs.
Learning capability - Agentic AI systems learn and improve as they work. Each interaction helps it get better at their tasks, using feedback and new data to refine their performance. It can switch tactics when things change.
But this independence brings up important questions. When AI systems can make their own decisions, who's responsible for their actions? How do we make sure they stay reliable and trustworthy? These are key issues teams need to consider when working with agentic AI.
Key differences between AI Agents and Agentic AI
Applications and use cases
AI Agents applications and use cases
Customer Support Smart chatbots now handle the front lines of customer service. They answer product questions, guide users through basic troubleshooting, and connect them to human agents when needed. They work within defined scripts but can handle a high volume of common requests 24/7.
E-commerce Systems Behind every "you might also like" suggestion is an AI agent processing your shopping patterns. These systems track browsing history, past purchases, and similar user profiles to suggest relevant products. They work from clear rules about what defines a good match.
Workflow Automation These agents tackle repetitive office tasks. They automatically sort incoming emails, process form submissions, generate standard reports, and flag items that need human review. Each agent focuses on a specific part of the workflow, following set rules to keep things moving.
Agentic AI applications and use cases
Healthcare Analytics These systems do more than just spot patterns - they connect dots across patient histories, lab results, and medical research. They can suggest unusual diagnosis paths human doctors might not consider, adapt their analysis as new data comes in, and learn from treatment outcomes to refine future recommendations.
Autonomous Logistics Smart robots in warehouses and delivery systems make real-time decisions. They plan optimal routes, avoid obstacles, work around equipment failures, and coordinate with other units. When they encounter new situations, they adapt their strategies rather than waiting for new programming.
Strategic Planning Systems These AIs help with complex business decisions by analyzing market trends, supply chain data, and competitor actions. They can propose multiple solutions, explain their reasoning, and adjust recommendations as conditions change. Unlike simpler analysis tools, they actively look for new factors that might affect outcomes.
AI agents and agentic AI aren't competing technologies - they're different tools for different jobs. When you need reliable, focused task handling, AI agents are your go-to choice. For challenges that need more flexible, independent thinking, agentic AI might be the answer.
The key is knowing what each one brings to the table. This helps you make smart choices about which technology fits your specific needs.