Boosted Prompt Ensembles for Large Language Models -Summary
The paper proposes a prompt ensembling method for large language models called 'boosted prompting', which uses a small dataset to construct a set of few shot prompts that together comprise a boosted prompt ensemble. The few shot examples for each prompt are chosen in a stepwise fashion to be 'hard'
Arxiv URL: https://arxiv.org/abs/2304.05970
Authors: Silviu Pitis, Michael R. Zhang, Andrew Wang, Jimmy Ba
What is Boosted Prompt Ensembling?
The paper proposes a prompt ensembling method for large language models called 'boosted prompting'. This method uses a small dataset to construct a set of few shot prompts that together comprise a boosted prompt ensemble. The few short examples for each prompt are chosen in a stepwise fashion to be 'hard' examples on which the previous step's ensemble is uncertain. The algorithm outperforms single-prompt output-space ensembles and bagged prompt-space ensembles on challenging datasets like GSM8k and AQuA.
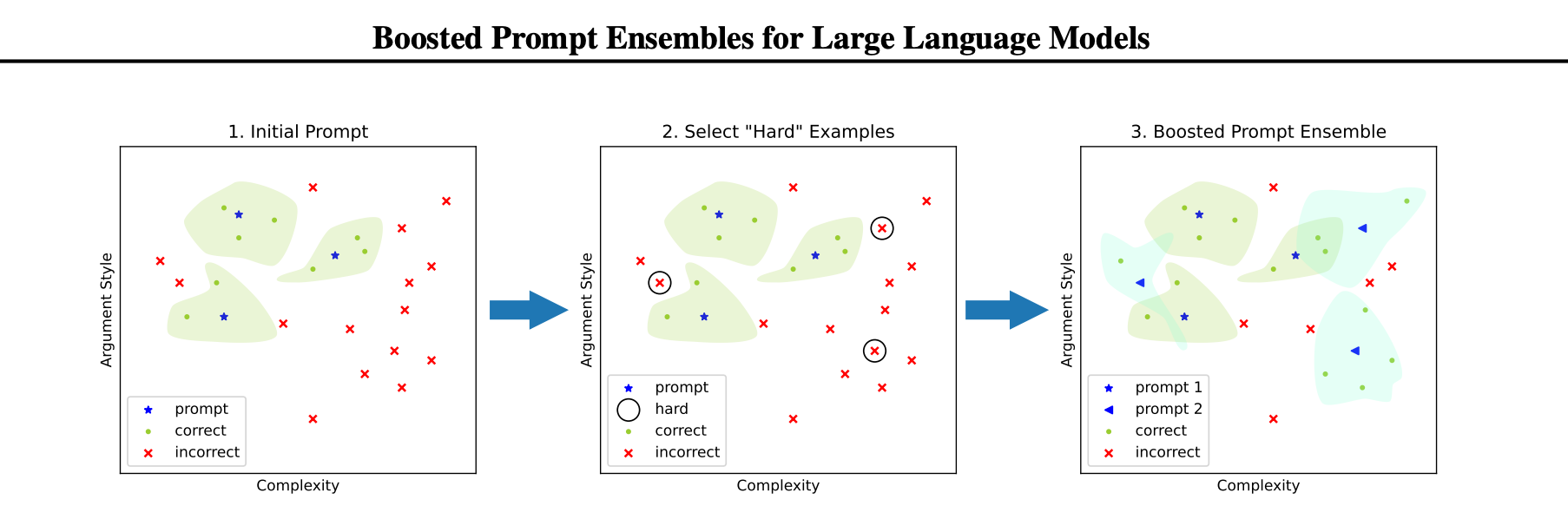
What are the key advantages?
- Improves performance over single-prompt and bagged ensembles
- Works with as few as 100 labeled examples
- Requires minimal manual prompt engineering
- Complements other reasoning techniques like chain-of-thought
- Can potentially adapt to distribution shifts in test-time variant
- Achieves strong results on multiple reasoning benchmarks
What are the limitations/disadvantages?
- Requires at least some labeled data for best performance
- Test-time version generally performs worse than the train-time version
- Performance depends on the quality of the initial prompt
- It may not help weaker language models (e.g., didn't improve Curie's performance)
- Computationally more expensive than single prompts
- Requires sufficient model accuracy to generate reliable self-supervision
What datasets was it tested on?
- AQUA (algebra questions)
- GSM8K (grade school math)
- MMLU570 (multi-task understanding)
- CMATH420 (competition math)
- SVAMP (arithmetic word problems)
What are the key insights & learnings?
- Boosted prompting outperforms single-prompt output-space ensembles and bagged prompt-space ensembles on challenging datasets like GSM8k and AQuA.
- The algorithm uses a small dataset to construct a set of a few shot prompts that together comprise a boosted prompt ensemble.
- The few shot examples for each prompt are chosen in a stepwise fashion to be 'hard' examples on which the previous step's ensemble is uncertain.
- The algorithm proposes both train-time and test-time versions of boosted prompting that use different levels of available annotation.
- The algorithm leverages a small dataset to construct a set of few shot prompts that progressively solve more of the problems, inspired by classical boosting algorithms.
Terms Mentioned: Large Language Models, few shot prompts, boosted prompting, output-space ensembles, bagged prompt-space ensembles, GSM8k, AQuA, train-time boosting, test-time boosting, annotation
Technologies / Libraries Mentioned: PyTorch, Hugging Face Transformers