The State of AI FinOps 2025: Key Insights from FinOps Foundation's Latest Report
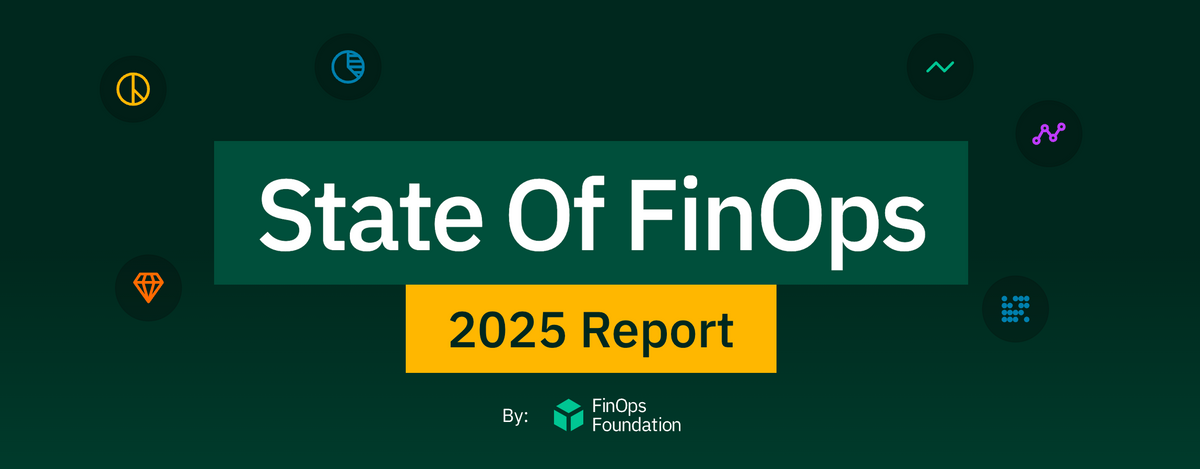
AI spending has doubled in enterprise environments, with a clear focus on establishing fundamentals before optimization. Dive into the latest FinOps Foundation report to understand how organizations are managing their AI infrastructure costs and what this means for your GenAI initiatives.
This is a summary blog focusing on AI trends from the FinOps Foundation's 5th Annual State of Finops survey
The FinOps Foundation just released their 5th Annual State of FinOps survey, analyzing over $69B in cloud spend across major enterprises. The findings reveal a dramatic shift in how organizations are approaching AI cost management and governance. Let's dive into the key insights and what they mean for teams building GenAI applications.
At Portkey, we work with over 650+ global organizations processing more than 25 billion LLM tokens daily, giving us a unique perspective on these evolving AI infrastructure challenges. The FinOps Foundation's findings strongly resonate with what we're seeing in the field.
Key Takeaways:
- AI cost management adoption doubled to 63% in 2024 (2x growth from last year)
- 97% of organizations investing across multiple infrastructure types
- Org focus is shifting from optimization to establishing fundamentals
The AI FinOps Explosion
The most striking finding from the report is the massive increase in AI cost management adoption. 63% of organizations now actively manage their AI spending, more than double from last year's 31%. This surge reflects the rapid mainstreaming of AI technologies and the growing recognition that effective cost management is crucial for sustainable AI adoption.
Investment Patterns: A Multi-Infrastructure Reality
Organizations aren't just increasing their AI investments – they're diversifying them across multiple infrastructure types. The report reveals that 97% of respondents are investing in AI across various infrastructure areas, with some interesting patterns emerging:
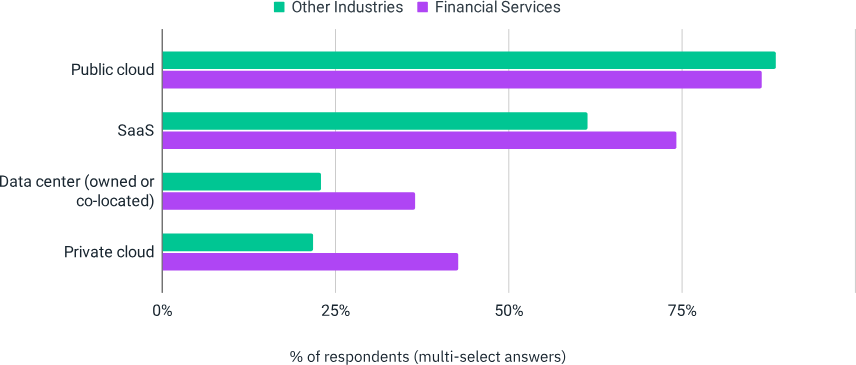
A few key observations:
- Public cloud remains the dominant platform for AI initiatives
- Financial services companies show notably higher investment in private cloud and data center solutions
- SaaS-based AI solutions are gaining significant traction across all industries
The Maturity Journey: Fundamentals First
One of the most insightful findings is how organizations are approaching AI cost management. Rather than jumping straight to optimization, they're focusing on establishing strong foundations:
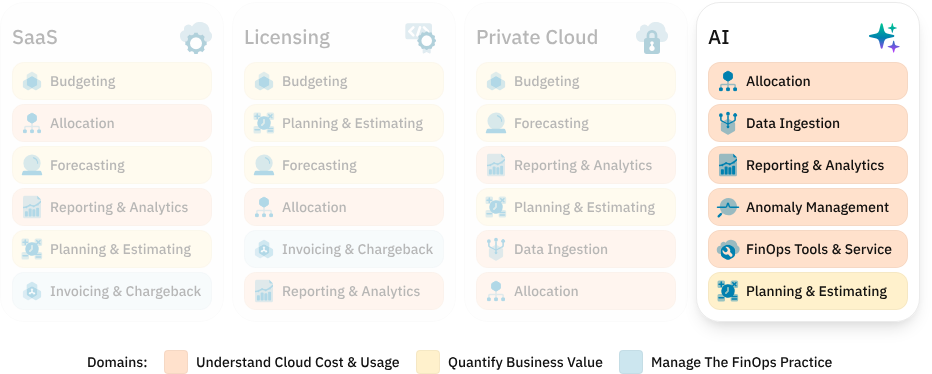
The top priorities fall under two key domains:
- Understanding Cloud Usage & Cost
- Quantifying Business Value
This focus on fundamentals before optimization mirrors the evolution we saw in cloud adoption. Organizations are recognizing that without proper visibility and allocation mechanisms, any optimization efforts will be built on shaky ground.
This maturity journey is something we've observed closely at Portkey. Organizations that successfully scale their AI initiatives typically start by establishing strong foundations in cost visibility and governance. We've seen teams achieve up to 75% faster time to market when they implement proper cost management and governance frameworks from the start.
Key Challenges and Priorities
The report highlights several critical areas that organizations are focusing on:
1) Cost Allocation and Chargebacks
Teams need to understand which departments and projects are driving AI costs. This becomes particularly complex with shared AI infrastructure and cross-functional use cases.
2) Data Ingestion and Processing
Understanding the full cost picture requires collecting and processing data from multiple sources – cloud providers, SaaS platforms, and on-premises infrastructure.
3) Reporting and Analytics
Organizations need comprehensive visibility into their AI spending patterns, usage metrics, and performance indicators to make informed decisions.
4) Anomaly Management
With AI workloads being more dynamic and potentially more expensive than traditional compute workloads, robust anomaly detection becomes crucial.
5) Planning and Forecasting
Teams need better tools and methodologies to estimate and plan their AI infrastructure needs and costs.
Bridging the Gap: From Challenges to Solutions
While these challenges might seem daunting, they're not insurmountable. For instance, at Portkey, we've helped teams implement solutions that address these exact pain points:
1) Cost Allocation & Chargebacks → Granular Attribution:
Real-world scenario: Imagine a large enterprise where multiple teams (Customer Support, Sales, and Product) all use AI for different purposes. Each team needs different models and has different usage patterns. With Portkey's granular cost attribution:
- Track costs down to individual prompts and model calls
- Automatically allocate costs to specific teams, projects, or cost centers
- Generate detailed chargeback reports for finance teams
- Set up team-specific budgets and alerts
2) Data Ingestion & Processing → Unified Data Pipeline
The challenge of collecting data from multiple sources (Azure OpenAI, AWS, OpenAI, etc.) is solved through:
- Automated data collection across all AI services
- Standardized metrics across different model types
- Real-time data processing for immediate insights
- Support for both cloud and on-premise LLMs
3) Reporting & Analytics → Comprehensive Dashboards
Portkey turns complex data into actionable insights:
- 40+ in-production metrics tracking both cost and performance
- Real-time visibility into spending patterns
- Custom reports for different stakeholders
- Trend analysis and usage patterns
4) Anomaly Management → Proactive Alerts
AI costs can spiral quickly without proper controls. Portkey addresses this through:
- Real-time budget tracking and alerts
- Automatic detection of unusual spending patterns, including:
- Sudden spikes in token usage that may indicate prompt injection attacks
- Unexpected increases in cost-per-request
- Abnormal patterns in model response times - Custom thresholds for different teams and use cases
- Immediate notifications when costs exceed set limits
- Automated actions to prevent runaway costs (e.g., rate limiting or routing to backup models)
5) Planning & Forecasting → Data-Driven Predictions
Portkey helps you make informed decisions about AI infrastructure with:
- Historical usage analysis for accurate forecasting
- Model-specific cost comparisons
- Capacity planning tools
- Budget optimization recommendations
Real-World Implementation Example
Let's look at how this works in practice with one of our enterprise customers:
A global technology company with 1000+ developers needed to manage their AI costs across multiple teams:
Marketing Team:
- Uses GPT-4o for content generation
- Monthly budget: $10,000
- Peak usage during campaigns
Engineering Team:
- Uses Claude for code analysis
- Monthly budget: $25,000
- Consistent daily usage
Research Team:
- Uses multiple specialized models
- Monthly budget: $15,000
- Project-based usage spikes
After implementing proper FinOps practices through Portkey:
- Each team got their own budget allocation with automated budget alerts that prevented any overruns
- Usage is tracked in real-time
- Implemented team-specific dashboards for AI usage that drove accountability
- Costs are automatically charged back to respective departments
Key Outcomes:
- Teams maintained high performance while identifying opportunities for cost savings
- Finance got accurate department-wise AI spending reports and could adjust budgets based on actual usage
- Engineering got real-time visibility into model performance and could help optimize spending across different models
- Leadership could make data-driven decisions about AI investments
What This Means for Your AI Initiatives
The report's findings suggest several key considerations for organizations building AI applications:
- Start with Strong Foundations: Focus on establishing solid cost visibility and allocation mechanisms before diving into optimization.
- Think Multi-Infrastructure: Your AI cost management strategy needs to account for workloads running across different infrastructure types.
- Invest in Automation: The complexity of AI cost management demands automated solutions for tracking, allocating, and optimizing costs.
- Focus on Business Value: While controlling costs is important, the primary focus should be on understanding and optimizing the business value generated from AI investments.
Looking Ahead
The report makes it clear that we're at an inflection point in AI adoption. As organizations move from experimental to production AI systems, the need for robust financial governance becomes critical.
The focus on fundamentals over pure optimization suggests that the market is maturing thoughtfully. Organizations are learning from the cloud adoption journey and applying those lessons to AI infrastructure management.
The path forward requires tools and platforms that can grow with your AI initiatives. For example, Postman, Clearcover, and multiple Fortune 500 companies have seen significant improvements in their AI operations - achieving 25x improvement in reliability and 90% faster deployments - by implementing robust governance and cost management frameworks through Portkey.
For teams building AI applications, the message is clear: invest in strong financial governance foundations now. The organizations that succeed in scaling their AI initiatives will be those that treat AI infrastructure as a strategic asset, with proper visibility, control, and optimization mechanisms in place.
Ready to establish strong FinOps practices for your AI initiatives? Learn how Portkey can help you implement these insights and accelerate your AI journey. Contact our team or sign up to Portkey here to get started.